Comparative analysis between AI CT imaging software and radiologists, nodule histology and/or patient clinical follow up
An independent, retrospective head-to-head comparative analysis of Artificial Intelligence-based medical software that detects pulmonary nodules in thoracic computed tomography (CT) scans retrieved from the 4-In-The-Lung-Run EU trial and other lung cancer screening trials.
Challenges
Several studies have assessed AI software packages for the detection of lung nodules in chest CT scans, showing good performance in detection, segmentation, and classification. In lung cancer screening (LCS), AI has demonstrated the potential to surpass radiologists in avoiding the misclassification of nodules. Specifically, AI can reduce the misclassification of nodules as seen in consensus readings. Additionally, AI could significantly reduce radiologists’ workload.
Despite the promising results of AI performance in LCS, real-world implementation and clinical adoption is slow. Even though there are numerous CE-marked AI products in radiology, the sector remains in its infancy, with a significant portion lacking peer-reviewed evidence of efficacy and only a few demonstrating potential clinical impact. Creating the first benchmark of AI CT software in Lung Cancer Screening would promote transparent development and implementation and provide purchasers with the evidence needed to make well-informed decisions.
iDNA’s Validation Platform
Professional standardized 4-phase comparison against leading AI companies
Benchmarking with high-quality lung cancer screening datasets based on several clinical, analytical and technical parameters
Datasets include manual reads from experienced EU radiologists with ground truth established through histopathological reports, including follow-ups
Personalized and applicable feedback per AI software package
Private cloud based installments and VPN-protected access to virtual environments at bn-infra, located in Groningen, the Netherlands
iDNA’s Infrastructure
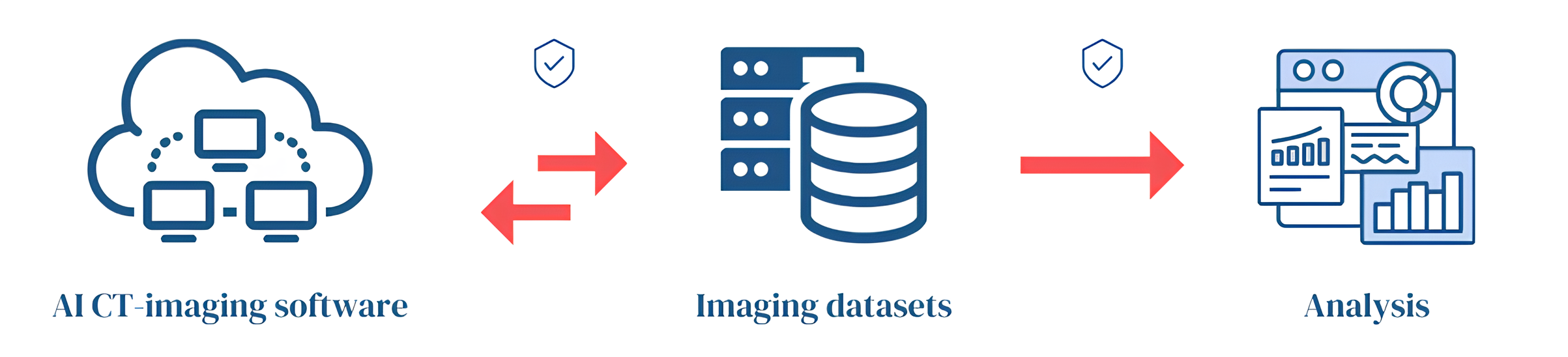
Interested in participating? Please contact us here
References
van Leeuwen, K.G., Schalekamp, S., Rutten, M.J.C.M. et al. Artificial intelligence in radiology: 100 commercially available products and their scientific evidence. Eur Radiol 31, 3797–3804 (2021). https://doi.org/10.1007/s00330-021-07892-z
Lancaster HL, Zheng S, Aleshina OO, Yu D, Yu. Chernina V, Heuvelmans MA, et al. Outstanding negative prediction performance of solid pulmonary nodule volume AI for ultra-LDCT baseline lung cancer screening risk stratification. Lung Cancer [Internet]. 2022 Mar 1 [cited 2023 May 8];165:133–40. Available from: http://www.lungcancerjournal.info/article/S0169500222000022/fulltext
European Commission. 4-IN THE LUNG RUN: towards INdividually tailored INvitations, screening INtervals, and INtegrated co-morbidity reducing strategies in lung cancer screening. Cordis Europa. 2022.
Field JK, Vulkan D, Davies MPA, Baldwin DR, Brain KE, Devaraj A, et al. Lung cancer mortality reduction by LDCT screening: UKLS randomised trial results and international meta-analysis. The Lancet Regional Health – Europe. 2021 Nov 1;10:100179.
Chiu HY;, Chao HS;, Petrella F, Chiu HY, Chao HS, Chen YM. Application of Artificial Intelligence in Lung Cancer. Cancers 2022, Vol 14, Page 1370 [Internet]. 2022 Mar 8 [cited 2023 May 8];14(6):1370. Available from: https://www.mdpi.com/2072-6694/14/6/1370/htm
Zheng S, Guo J, Cui X, Veldhuis RNJ, Oudkerk M, Van Ooijen PMA. Automatic Pulmonary Nodule Detection in CT Scans Using Convolutional Neural Networks Based on Maximum Intensity Projection. IEEE Trans Med Imaging. 2020 Mar 1;39(3):797–805.